By following industries such as Aviation, Travel, and Hospitality find that customer journeys are crucial to their business. They have to deploy sentiment analysis to customer reviews, prompt feedback, and brand perception sessions. In recent times, businesses have invested huge infrastructure costs to improve better-managed customer experiences. In addition to the separation of positive and negative emotions, there has been an emergence of a second scale between objectivity and subjectivity.
However, the main aim of sentiment analysis is to detect the differences between the objective and subjective areas, there are efforts to identify encompassing emotions such as anger, enjoyment, and fear. The other aim is to improve the system’s accuracy and advocacy. There are many benefits to using sentiment analysis such as aiding decisions, adopting new products in the markets, or simply monitoring brands.
Understanding the crucial role of Sentiment Analysis
Sentiment analysis brings analyzing the sentiment or opinion expressed in a given text to the front view. The main task is to analyze the conflict of the text, whether it is positive, negative, or neutral. In addition, The categorization within the text can be implemented at file, stance, and specific aspects level. Sometimes the sentiment analysis can go beyond just polarity and considers feelings such as enjoyment, anger, disgust, sadness, fear, and surprise.
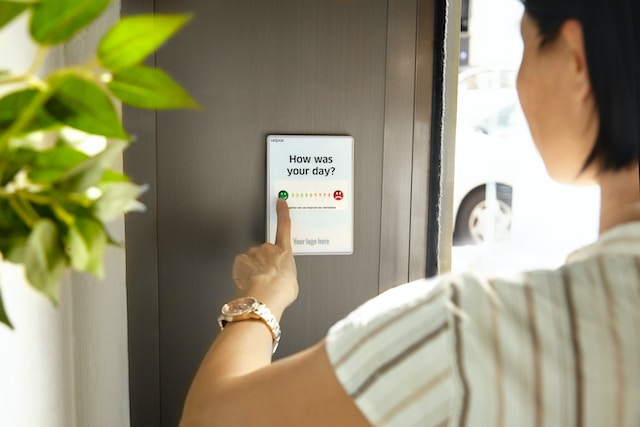
It is important to determine whether your customer feedback is objective or subjective
The subjectivity of words and phrases can vary depending on their context. Even an objective document may contain subjective sentences, such as a news article quoting people’s opinions. Research has shown that removing objective sentences from a document before classifying its polarity can improve the performance of sentimental analysis systems. It is crucial for the system to be able to identify and separate objective and subjective contexts within the text, as there may be a mixture of both.
Applications of Sentiment Analysis
The best way sentiment analysis shows its impact is through the inward view of how the business is noticed and how the business’s strategies affect the profitability of new product offerings and customer perception. In a specific case study, sentiment analysis can be used by customer service personnel to segregate and prioritize their customer service-related communication into different buckets so as to address top priority ones at lightning speeds. This automation can assist the personnel to mitigate critical customer service issues.
With business intelligence initiatives, customers’ pain points can be studied and probed further through subjective reasoning on their actions. Furthermore, finding the cause of such actions can be investigated and certain trends can be brought forward.
Exploring the varied approaches to Sentiment Analysis
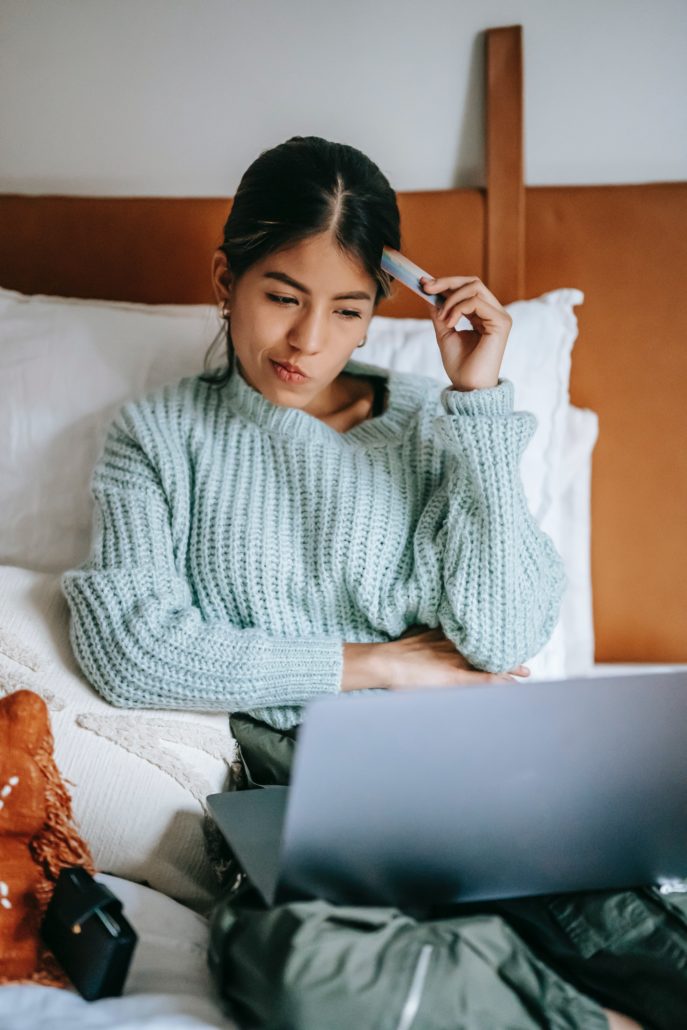
The main approach to sentiment analysis branches out from two main nodes
When using knowledge-based techniques, text is classified based on the presence of an unambiguous array of happy, sad, afraid, and boring words. When using statistical-based techniques, it is conducted by analyzing giant data fields such as machine learning and big data discipline. However, the hybrid approaches leverage both machine learning and elements from knowledge representation such as ontologies and semantic networks in order to detect semantics that are expressed in a subtle manner, e.g., through the analysis of concepts that do not explicitly convey relevant information, but which are implicitly linked to other concepts that do so.
The analyses offer airlines prompt information and allow them to make prompt decisions to streamline their product line. However, it might allow the decision-makers to quickly throw and burn failing product ideas. Based on the decision factors that the approaches offer, they can be used as a base to improve customer experience.
Unveiling The Process of Sentiment Analysis
Sentiment analysis, which is also known as opinion mining, is used to determine the sentiment or emotional tone expressed from a string of text. By analyzing the text string, you can determine whether it is a positive, negative, or neutral sentiment. This allows Sentiment analysis to be used on a range of use cases such as customer reviews, social media posts, and many more. When we classify text, we put this in different categories based on the sentiment such as positive, negative, or neutral. Using machine learning techniques, algorithms such as Naive Bayes, Support Vector Machines, etc can be used to train models and systems based on data to predict the sentiment. With the Lexicon-based approach, pre-defined dictionaries or lexicons containing words or phrases associated with pre-defined sentiment directions are used to determine the sentiment of a given text.
The overall filter to sentiment analysis is aspect-based, where this approach mainly focuses on analyzing sentiment towards specific aspects or features that are chosen to be the target focus to focus more small but powerful insights For more accurate and focused results, the scope of domain-specific sentiment analysis is more useful as it can be tailored towards a specific domain or industry.
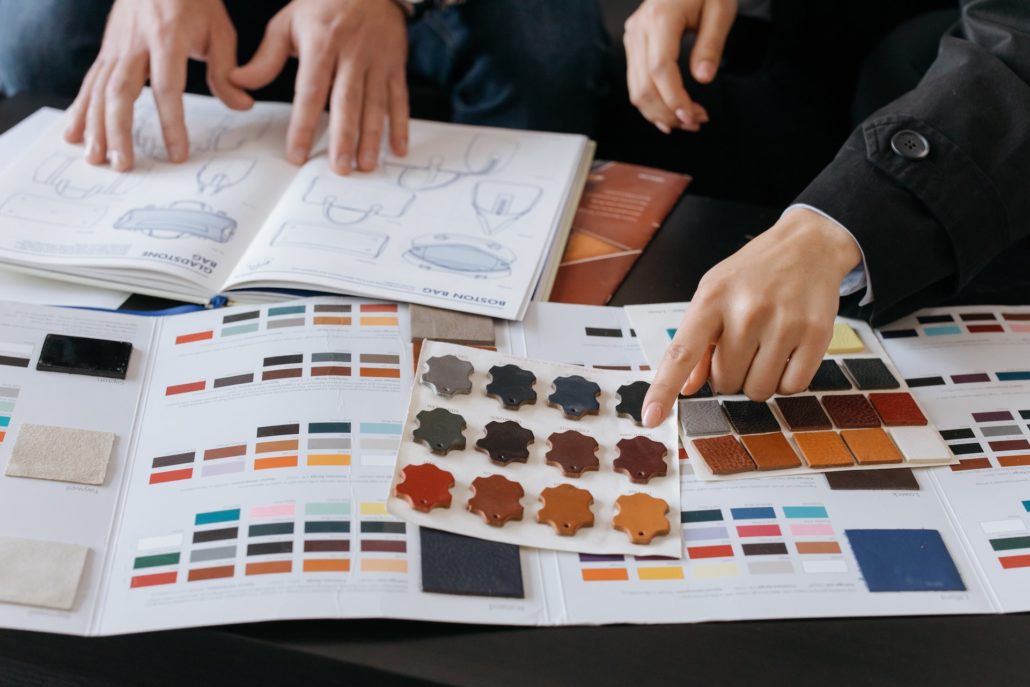
Learn new patterns from your customers
Branching out from machine machine-based method, the difference between supervised and unsupervised learning method is that in the supervised method the data and results is observed and labeled under pre-defined norms. However, the use of semi-supervised methods is recommended (Xianghua et al., 2013) as it is difficult to rely completely on supervised methods with an initial group of tagged documents to group others. The combined use of supervised and unsupervised methods is useful for categorizing sentiments.
Following the lexicon-based method, there are the dictionary and corpus-based approaches where after the search of seed words is requested, the comparison of synonyms and antonyms starts with building word lists for each word. Corpus-based approaches bring out the polarity of the words through a combination of seed words and opinions built through a series of listing techniques. The latter is more agile, as the need for unnecessary data is not needed, as the system reaches its useful stage quickly through its domain-related corpus.
Furthermore, there are many features that promote the usefulness of sentiment analysis such as
- feature extraction – This cleans up the unnecessary feature not required for data analysis
- sentiment intensity – Determining the intensity of the sentiment can show another dimension of polarity in the feedback text.
- sentiment visualization – the sentiment can also be displayed in the form of heat maps and shown as a clear presentation of how the sentiment is distributed.
- sentiment monitoring – the choice of tools required for monitoring is critical as every business is different and the need to track and respond to customer feedback is important.
- sentiment analysis can have different applications within the same industry. This can cater to each segment of the same department such as brand monitoring, social media monitoring, market research, etc
Elevating Tourism Using Sentiment Analysis
However, it is a difficult process to reveal emotional expressions in such a huge amount of data. In many cases, sentiments are hidden in the huge and natural language content produced by customers. The main approach to sentiment analysis of touristic products is based on determining the sentiment polarity in customer reviews. To fully understand and conduct an effective sentiment analysis in the tourism industry, the system has incorporated a natural language process, text mining, and a stringent sentiment analysis process.
Direct selling has proven to be effective in controlling the perception by tourists of being of high value. Strangely when tourism products and services are sold through a third party or as part of a packaged deal, tourists suspect a lesser value of a product being offered to them. Tourism suppliers have an upper hand in evaluating their products and services through the valuable feedback they receive from the tourists themselves. Furthermore, the suppliers are in the position to amend their offerings to pivot towards the favor of the tourists, making them more attractive.
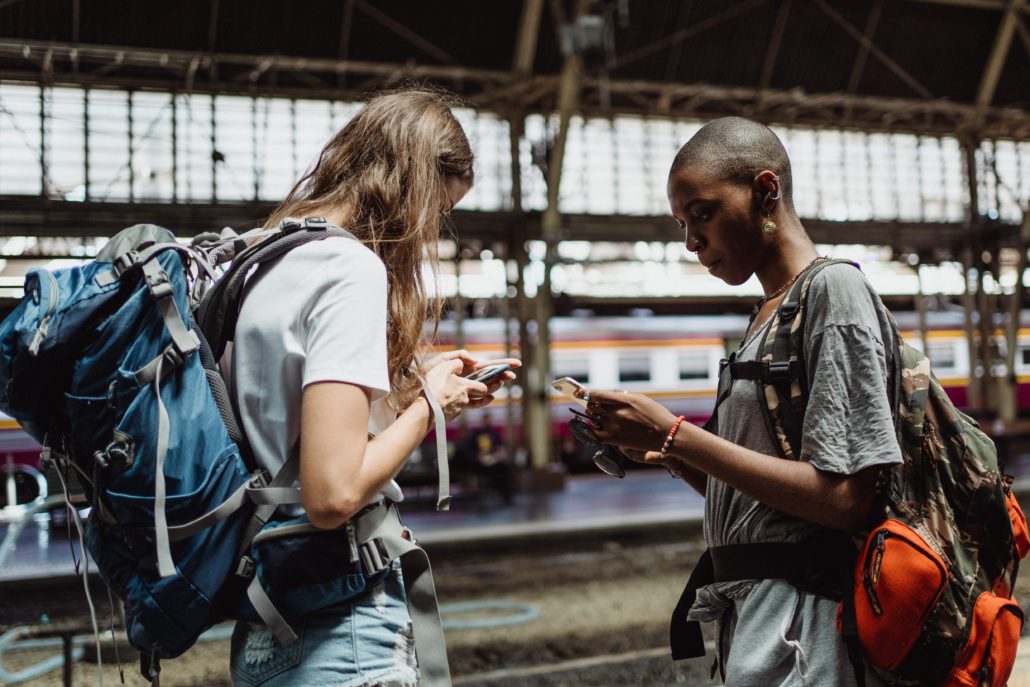
Customers will make their own opinions based on others
Building on the experience of another tourist, new travelers can choose their experience from stories shared on social media, blogs, forums, etc. Although tourists share their feedback effortlessly and without coercion, it is vital to have opportunities available for tourists to give their honest feedback. This would make it easier for the tourism business and tourist operators to collect feedback from tourists.
Preparing this data for special sentiment analysis programs to ingest and make sense of it. Most of the time, unstructured data is collected at this stage where basic tools like web scrapers and crawlers are used from review websites and other marketplaces. Tools that can convert this data into a structure by applying text mining and preprocessing techniques can prove to be valuable, as they process them into structured data, ready for sentiment analysis. This is critical, as data quality fed into the analysis will affect the outcome results. Once sentiment analysis is applied to the structured data. The respective sentiments about the business and its offerings are drawn out which are based on subjective and objective finding analysis. Finally, these findings and result outcomes are present through visualization tools and various conclusions can be derived and worked on based on the industry standards, reports, and business fairing.
Taking off and looking for opportunities onboard
The most common method of data collection in the aviation industry is Passenger Feedback. This is conducted through various methods such as customer reviews on airline websites, passenger opinions on the airline marketplace, traveler feedback, and satisfaction forms. Nowadays with the adoption of technology, this task is easier, which has led to the airline throwing out more surveys than before. This in a way has affected the customer experience by being interrupted frequently. The best way would be to allow the customer to share their feedback at their own time and encourage the staff to record feedback from customers based on their observations.
Airlines need to investigate their customer engagements and seek to involve the airline brand in important customer interactions which may lead to heightening their brand equity standing. Most airlines measure their reputation through brand sentiment, public perception, airline image through social media listening, reputation management measures, and customer sentiment analysis. It is evident that most flight reviews and passenger comments through ratings can shed some light on specific product launches and service offerings.
Most airlines have adopted technology into their service delivery methods to record customer preferences along with direct feedback. This has pushed cost-effective and waste management initiatives into the airline catering segments. The strategy professionals working under those portfolios have used sentiment analysis on big data collection through meal orders and preferences recorded inflight to carefully predict future catering loads with the intention of reducing wastage.
By using sentiment analysis, airlines can understand their competition much better, through benchmarking and comparison methods. Simply by performing sentiment analysis on their competitors, most airlines can draw out their understanding of their competitive advantage and better focus their strategy.
Challenges and Issues
The concept of big data is often used as an end-all solution towards achieving the optimal sentiment analysis platform and simply where the outcome can be derived from. However, as previously discussed the data that is fed in matters a lot in determining the outcome. The concern with big data is that it is mostly unstructured which doesn’t have a lot of use or purpose.
With the new buzz around ‘big data’, companies rush to collect the mammoth pile of data under the pretext of pursuing a data-driven approach to fulfill an empty mission. This fallacy of action steps is only undertaken by operation and customer-facing staff. However, the main heavy lifting is done by those who analyze, clean data to provide structure and visualize the outcome. The outcome of sentiment analysis is directly dependent on the medium of the input and the correlation with another dataset that affects operations such as weather, staff union strikes, aircraft availability, etc. The aviation industry enjoys the multitude of data points where it can receive feedback.
Opinion mining provides the outcome results in just 3 labels, such as positive, neutral, and negative. This limits the initiative that the business can pursue to complete its business objectives to find innovative ways to improve. Moreover, the aspect-based sentiment analysis gives a detailed output to draw a more realistic picture where the true nature of the current state along with the context is captured and fed into the analysis only to give a more accurate sentiment quotient. This is beneficial to the business to take more calculated action and swiftly deal with the root cause of problems that they are facing.